News & Articles
Modeling SARS-CoV-2 outbreaks in workplaces, universities, and other congregate settings
Julian Homburger
The SARS-CoV-2 pandemic has upended the way we work, teach, and socialize. As the pandemic continues, institutions are solving for how to bring people back on-site safely. One of the most important aspects of returning on-site is ensuring that adequate steps are taken to protect those who return on-site (and their families) from outbreaks of SARS-CoV-2. However, it is clear that our current approach of testing only symptomatic individuals is failing, and many outbreaks have been associated with reopening workplaces and schools. In contrast to our current approach, a proactive testing strategy, which involves testing all individuals within a population at a regular cadence regardless of symptoms, is a powerful tool that can successfully mitigate and contain outbreaks of SARS-CoV-2.
To better understand the ways in which proactive testing can mitigate outbreaks, we have worked extensively with Carl Bergstrom and Ryan McGee, experts in epidemiological modeling. We use their SEIRS+ model to understand SARS-CoV-2 disease dynamics and the effect that proactive testing can have on containing outbreaks. While many modeling efforts have focused on national or regional populations, here we focus on smaller populations, such as a workplace or university, that are often made up of groups of 50 to 1000 people. Based on our work, we have released an updated return-to-onsite protocol as well as an interactive web application of the results.
Our work clearly demonstrates:
– Frequent proactive viral testing for SARS-CoV-2 can contain outbreaks in these types of smaller populations.
– Fast test turnaround time is key to enable quick isolation of individuals infected with SARS-CoV-2, and that a turnaround time of 5 days or higher will eliminate much of the benefit of proactive testing.
The SEIRS+ Model
The SEIRS+ model is what epidemiologists call a compartmental model, in which an individual’s disease state is tracked over time. The basic disease states, or compartments, included in this type of model are:
– Susceptible – these are people who could be infected by the disease, but are not currently infected
– Exposed – these are people that have contracted the virus, but are not currently infectious
– Infectious – these are people who are infected with the virus and can infect others
– Recovered – these are people who are no longer infectious. While the type of immunity and protection from SARS-CoV-2 reinfection is unclear at this time, for the purposes of this model we assume recovered individuals are immune from reinfection.
Over time as the disease progresses, people move from one state to another with a certain probability, based on current understanding of the disease. Basic SEIR models have only the four compartments above. The SEIRS+ model is more detailed in that the infectious (I) compartment is divided into three different states: pre-symptomatic, asymptomatic, and symptomatic – an important detail considering the biology of Covid-19, where much of the spread of infection is from individuals who are not currently experiencing major symptoms. In addition, the SEIRS+ model allows us to move individuals into ‘quarantine’ states, in which individuals are removed from the population and so that they no longer contribute to the spread of the infection. This simulates the effects of testing and subsequent isolation of individuals who receive a positive test result. Below is a diagram of the different state changes included in our model, with arrows showing the movements an individual can make. Disease states include susceptible (S), exposed (E), the presymptomatic state (PsI), asymptomatic (AsI), symptomatic (SyI), and recovered (R). If an individual tests positive, they are isolated and move to one of the quarantine states (QPI, QAI, QSI, and QR) for 14 days.
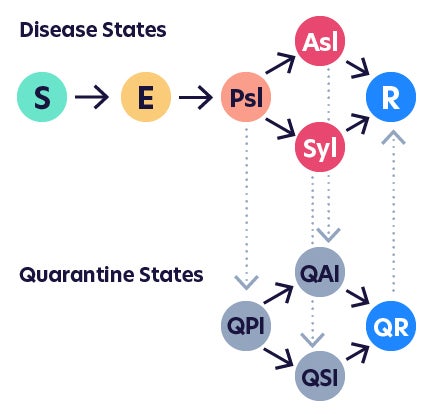
The SEIRS+ model also uses a network approach to simulate social contact networks. For example, in an office setting, a person is likely to have more meetings and contact with members of their team. The SEIRS+ model allows us to incorporate these types of team structure dynamics into the model and simulate close contacts being more likely to transmit the virus to each other.
Finally, the SEIRS+ model is a stochastic model. This means that simulations incorporate random chance into determining the course of the outbreak. For example, some individuals may infect more people than others, or take longer to recover from the disease. The SEIRS+ model incorporates these effects as randomness: at each point in time, it randomly chooses (based on event probabilities) what the next event (ie. infection, recovery – essentially any arrow in the figure) should be. Therefore, we perform 1000 simulations for each scenario to better understand the range of possible outcomes.
The SEIRS+ model code is freely available on their Github repository, and our modeling code can be found in our fork of their repository.
Exploring and Visualizing a Simulation
To help visualize these results, we’ve released a simple web application here. This webapp helps evaluate the impact that proactive testing and test turnaround time has on outbreak size, in different populations under variable conditions.
The model allows us to understand the effects and spread of the virus over time, starting with a single introductory infection. Each model run uses stochastic simulations to represent the outcomes of introducing a single SARS-CoV-2 case into the population over time. As an example, below is a plot of the outcomes of 50 simulations where a single case was introduced into a population of 1000 people without any interventions. Each line on the left tracks the current number of individuals infected or quarantined at any given point in time. In the top right is a smaller plot of outbreak time vs. total number of infections, demonstrating two different clusters of infection types. In this scenario, there is a huge amount of variability across simulations and a bimodal distribution of the total number of infections. In many simulations introductions don’t ‘spark’ an outbreak and die out – but other times they take-off, and lead to large-scale outbreaks, producing a bimodal distribution of outcomes.
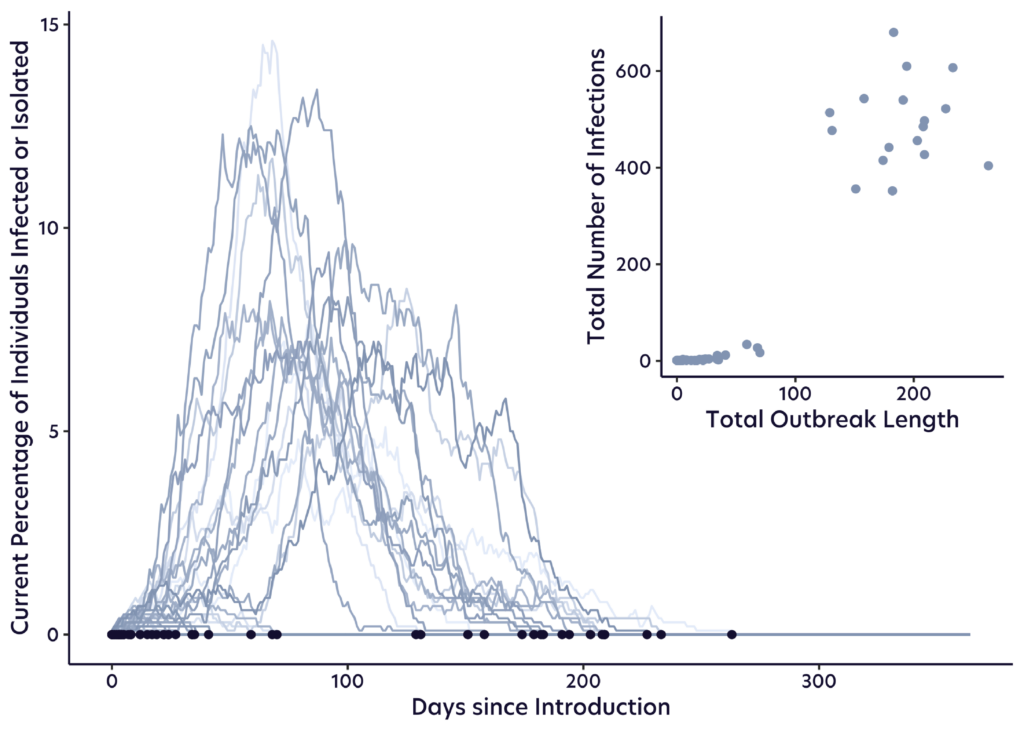
In order to understand the impact that testing could have on outbreaks in this population, the above simulation was repeated using the same assumptions and incorporating the use of weekly proactive testing as an intervention. The results are shown in the plot below, which includes the results from the ‘No Testing’ simulation in the top right panel for comparison. When weekly proactive testing was included in the model, fewer outbreaks occurred and those that did were contained early and quickly, infecting fewer individuals. This demonstrates the value of using proactive testing to identify and isolate positive cases before they infect others.
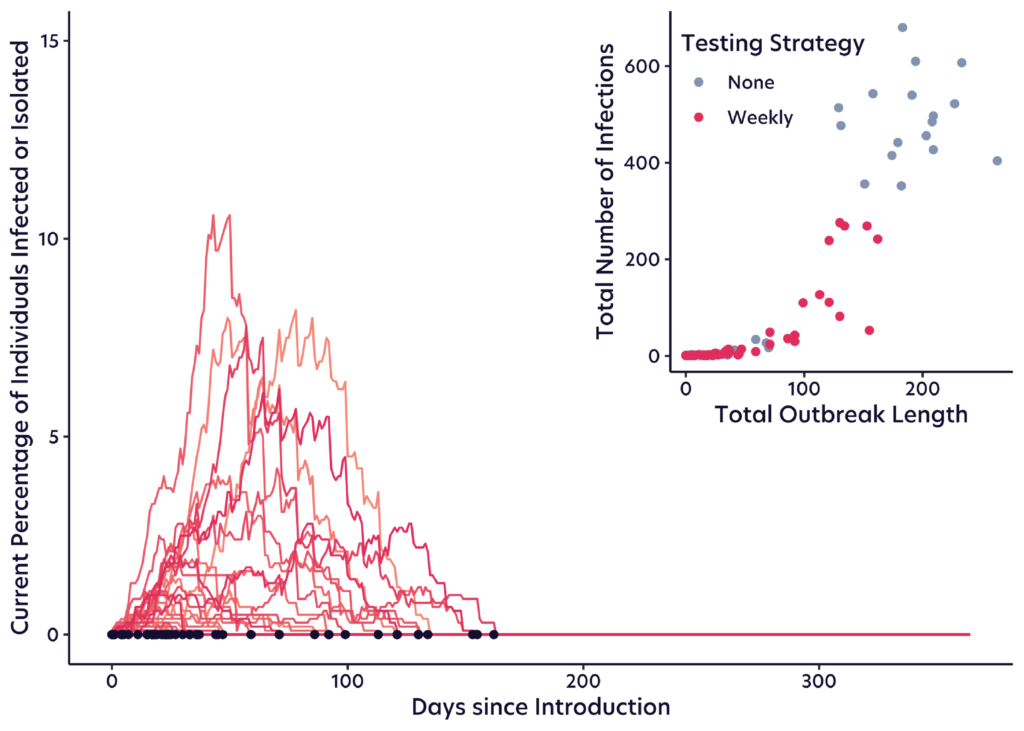
Comparing different scenarios and outcomes
In order to investigate more deeply the effects of testing cadence and turnaround time, we walk through a few example cases.
Building plots of the inverse cumulative distribution of total individuals infected for each model helps to better compare multiple models. The line for each model where the x value is the percentage of the population infected, and the y value is the percentage of simulations where x or more individuals were infected. What this means is that better outbreak mitigation measures lead to curves that become lower more quickly, while the bimodal distributions we saw with no testing lead to curves that have large flat lines. First, below is a comparison of no testing, weekly, and semi-weekly testing. A curve that decreases more quickly is more favorable – this means that fewer individuals were infected in a higher proportion of our simulations. We see that, as expected, more testing can reduce the size and chance of an outbreak.
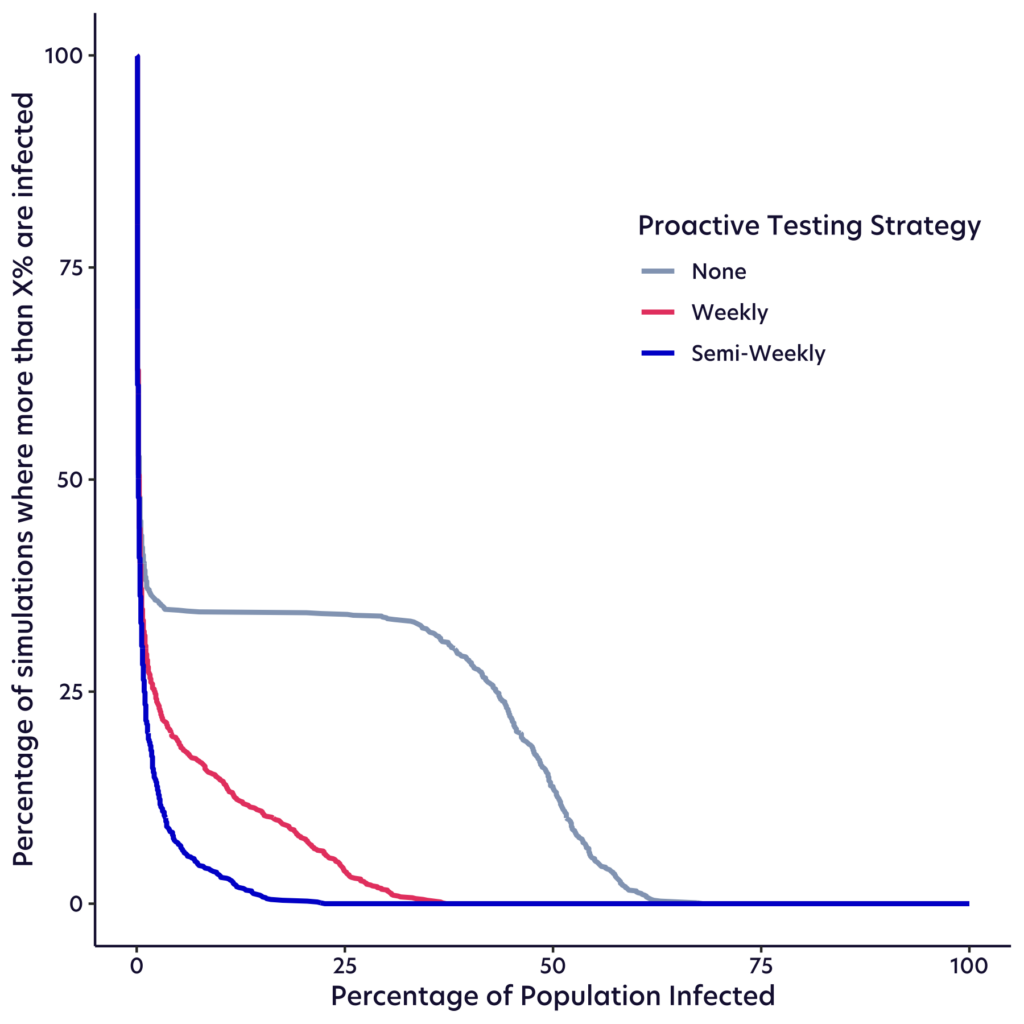
Test turnaround time is another area of significant importance for testing laboratories. The model shows that testing less frequently with a fast turnaround time performs better than testing more frequently but having to wait for results. The figure below demonstrates that when test turn-around time moves from 1 days to 5 days, even when testing as frequently as twice a week, the chance of a large outbreak (greater than 5% infected) increases from just 1.4% to 26.8%. This suggests that returning results to participants quickly is essential for proactive testing strategies.
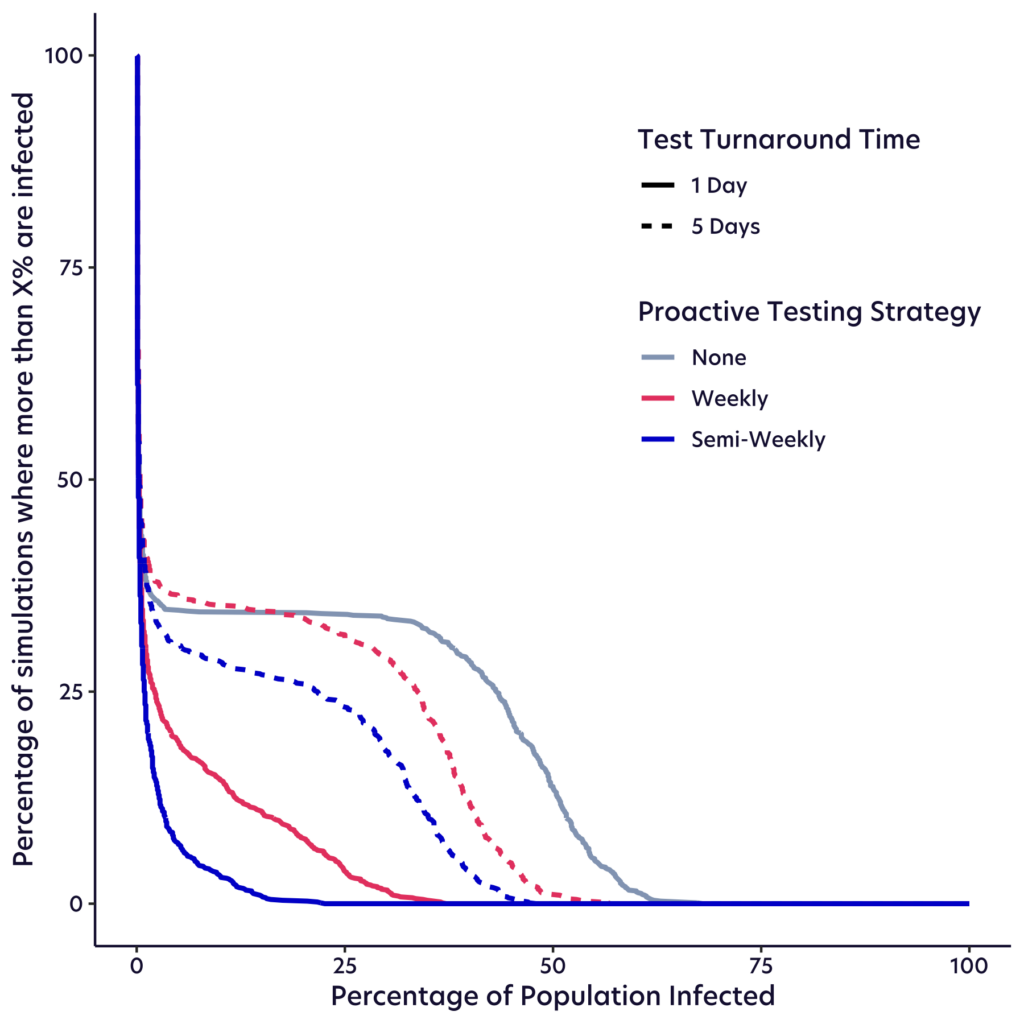
We are excited to release the results of our models in a web application that allows viewers to explore a range of different scenarios. The SEIR+ model can provide insights into different mitigation measures and outbreak probabilities under many diverse scenarios. We hope that it helps individuals to better understand the role that proactive testing can have in controlling outbreaks of SARS-CoV-2.
About Color
Color is a leader in distributed healthcare and clinical testing. Color makes population-scale healthcare programs accessible, convenient, and cost-effective for everyone. Color works with health systems, employers, and national health initiatives around the world including the million-person All of Us Research Program by the National Institutes of Health. For more information about Color and its response to COVID-19, visit www.color.com/covid-contact.